Optimal Blog
Articles and Podcasts on Customer Service, AI and Automation, Product, and more
.png)
We’re excited to launch our video recording functionality for prototype testing, enabling you to dive deeper into the “why” behind user actions and empowering you to make data-informed decisions faster and with greater confidence.
See User Actions Come to Life
Capture the nuance of user interactions with screen, audio, and/or video recording. With Optimal’s video recording feature, you can:
- Understand Intent: Watch users in action to reveal their decision-making process.
- Spot Friction Points: Identify moments of hesitation, confusion, or frustration.
- Test Your Ideas: Leverage user insights to make informed decisions before moving forward.
- Track Task Success: Combine video insights with quantitative data to understand what works and what needs refinement.
- Share Compelling Insights: Use recordings to drive alignment across your team and key stakeholders.
Drive Value with Video Recordings and Prototype Testing
By combining video recordings with prototype testing, you can unlock actionable insights that make a real impact.
Here’s how they drive value for your initiatives:
- Higher Conversion Rates: Optimized designs based on real user feedback lead to increased engagement.
- Greater User Satisfaction: Tested prototypes help to better align your experiences with user needs and expectations.
- Reduced Development Costs: Catch issues early to avoid costly fixes later in the development process.
- Faster Time-to-Market: Resolve design flaws early to accelerate project timelines.
Recruit the Right Participants for Richer Results
Optimal combines the power of video recording, participant recruitment, and a comprehensive UX insights and research platform to elevate your product and research process.
Use Optimal’s recruitment service to quickly connect you with millions of people in 150+ countries ready to take part in your study. Our in-house team handles feasibility assessments, sends reminders and confirmations, reviews personalized study setups, and conducts human checks to ensure high quality participants to maximize the value of your video recordings.
Thank you, Beta Testers
We’re grateful to our early adopters and beta testers for shaping the future of video recording and prototype testing. Based on your valuable feedback, we’ve made the following updates:
Video recording updates
- Additional recording controls: You can now control whether to reject participants or forward a participant to a non-recording study link if they do not meet your recording criteria.
- Translations: Set your study language and translate the recording instructions into 180+ languages.
- No video expirations: We’ve removed video expirations, ensuring your recordings remain accessible as long as you have an active Optimal subscription.
- Improved participant experience: We’ve improved the technology to reduce technical errors, creating a more reliable and user-friendly experience.
Prototype testing updates
- Collapse/expand and move tasks: Increase prototype visibility by hiding or moving tasks, making it easier for participants to view and interact with more of your design, especially for mobile prototypes.
- Option to end tasks automatically: When enabled, tasks will automatically end 0.5 seconds after a participant reaches a correct destination, removing the need for participants to confirm that they've completed the task. This can improve the overall participant experience, removing steps and making tests faster to complete.
- Increased Figma frame limit: We’ve increased the Figma frame limit from 30 to 100 frames to support larger, more complex prototypes.
- Expanded task results: Task path results now indicated completed and skipped tasks for better analysis.
- Time-saving improvements: Auto-select the starting screen after importing a Figma prototype, and enjoy task selection persistence across tabs in the analysis view.
- Enhanced security: We’ve updated Figma authorization for expanded security for your prototypes.
Ready to unlock the power of video recording?
Get started with a prototype test in Optimal or visit our help documentation to learn more.
Topics
Research Methods
Popular
All topics
Latest
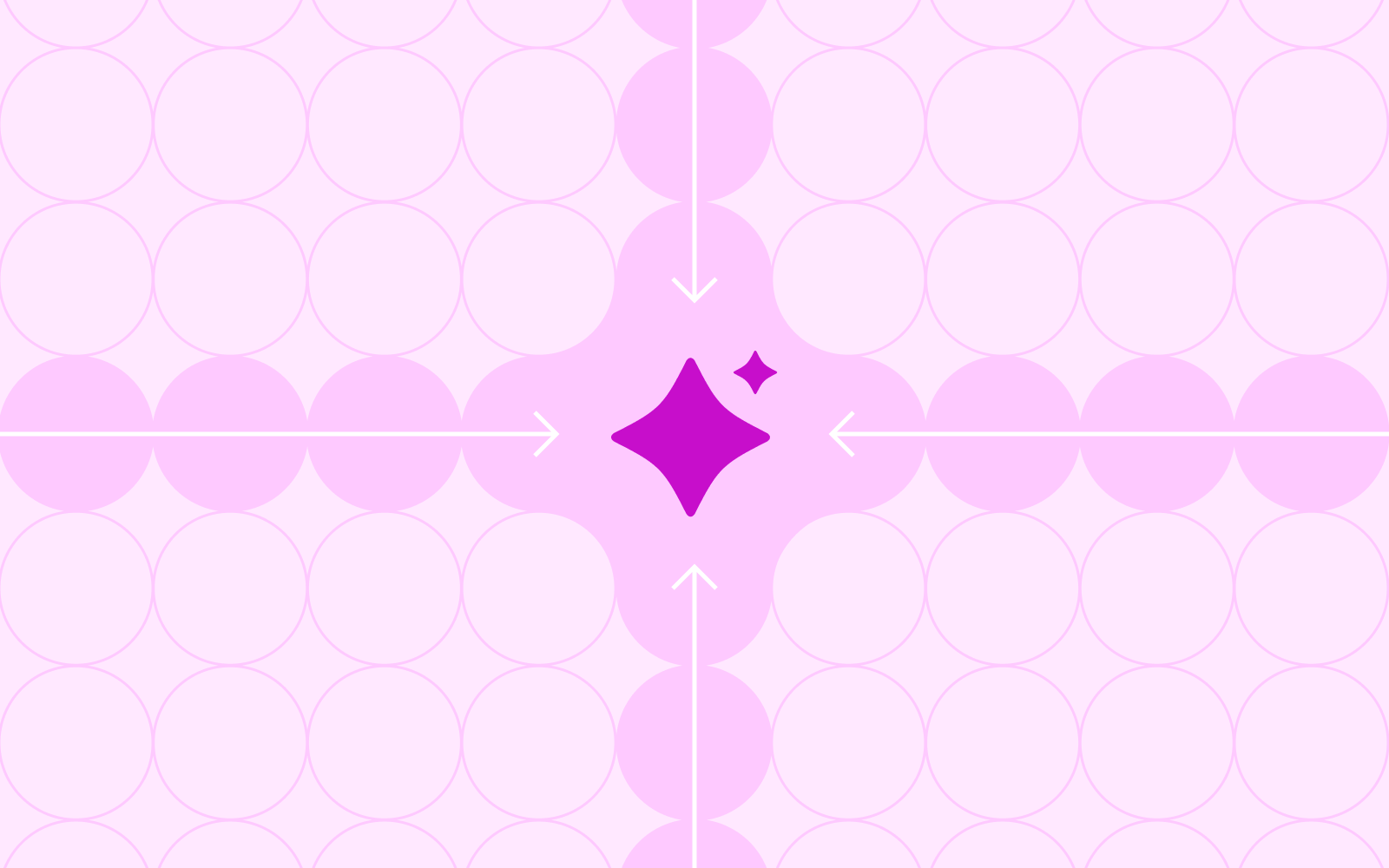
Designing User Experiences for Agentic AI: The Next Frontier
Beyond Generative AI: A New Paradigm Emerges
The AI landscape is undergoing a profound transformation. While generative AI has captured public imagination with its ability to create content, a new paradigm is quietly revolutionizing how we think about human-computer interaction: Agentic AI.
Unlike traditional software that waits for explicit commands or generative AI focused primarily on content creation, Agentic AI represents a fundamental shift toward truly autonomous systems. These advanced AI agents can independently make decisions, take actions, and solve complex problems with minimal human oversight. Rather than simply responding to prompts, they proactively work toward goals, demonstrating initiative and adaptability that more closely resembles human collaboration than traditional software interaction.
This evolution is already transforming industries across the board:
- In customer service, AI agents handle complex inquiries end-to-end
- In software development, they autonomously debug code and suggest improvements
- In healthcare, they monitor patient data and flag concerning patterns
- In finance, they analyze market trends and execute optimized strategies
- In manufacturing and logistics, they orchestrate complex operations with minimal human intervention
As these autonomous systems become more prevalent, designing exceptional user experiences for them becomes not just important, but essential. The challenge? Traditional UX approaches built around graphical user interfaces and direct manipulation fall short when designing for AI that thinks and acts independently.
The New Interaction Model: From Commands to Collaboration
Interacting with Agentic AI represents a fundamental departure from conventional software experiences. The predictable, structured nature of traditional GUIs—with their buttons, menus, and visual feedback—gives way to something more fluid, conversational, and at times, unpredictable.
The ideal Agentic AI experience feels less like operating a tool and more like collaborating with a capable teammate. This shift demands that UX designers look beyond the visual aspects of interfaces to consider entirely new interaction models that emphasize:
- Natural language as the primary interface
- The AI's ability to take initiative appropriately
- Establishing the right balance of autonomy and human control
- Building and maintaining trust through transparency
- Adapting to individual user preferences over time
The core challenge lies in bridging the gap between users accustomed to direct manipulation of software and the more abstract interactions inherent in systems that can think and act independently. How do we design experiences that harness the power of autonomy while maintaining the user's sense of control and understanding?
Understanding Users in the Age of Autonomous AI
The foundation of effective Agentic AI design begins with deep user understanding. Expectations for these autonomous agents are shaped by prior experiences with traditional AI assistants but require significant recalibration given their increased autonomy and capability.
Essential UX Research Methods for Agentic AI
Several research methodologies prove particularly valuable when designing for autonomous agents:
User Interviews provide rich qualitative insights into perceptions, trust factors, and control preferences. These conversations reveal the nuanced ways users think about AI autonomy—often accepting it readily for low-stakes tasks like calendar management while requiring more oversight for consequential decisions like financial planning.
Usability Testing with Agentic AI prototypes reveals how users react to AI initiative in real-time. Observing these interactions highlights moments where users feel empowered versus instances where they experience discomfort or confusion when the AI acts independently.
Longitudinal Studies track how user perceptions and interaction patterns evolve as the AI learns and adapts to individual preferences. Since Agentic AI improves through use, understanding this relationship over time provides critical design insights.
Ethnographic Research offers contextual understanding of how autonomous agents integrate into users' daily workflows and environments. This immersive approach reveals unmet needs and potential areas of friction that might not emerge in controlled testing environments.
Key Questions to Uncover
Effective research for Agentic AI should focus on several fundamental dimensions:
Perceived Autonomy: How much independence do users expect and desire from AI agents across different contexts? When does autonomy feel helpful versus intrusive?
Trust Factors: What elements contribute to users trusting an AI's decisions and actions? How quickly is trust lost when mistakes occur, and what mechanisms help rebuild it?
Control Mechanisms: What types of controls (pause, override, adjust parameters) do users expect to have over autonomous systems? How can these be implemented without undermining the benefits of autonomy?
Transparency Needs: What level of insight into the AI's reasoning do users require? How can this information be presented effectively without overwhelming them with technical complexity?
The answers to these questions vary significantly across user segments, task types, and domains—making comprehensive research essential for designing effective Agentic AI experiences.
Core UX Principles for Agentic AI Design
Designing for autonomous agents requires a unique set of principles that address their distinct characteristics and challenges:
Clear Communication
Effective Agentic AI interfaces facilitate natural, transparent communication between user and agent. The AI should clearly convey:
- Its capabilities and limitations upfront
- When it's taking action versus gathering information
- Why it's making specific recommendations or decisions
- What information it's using to inform its actions
Just as with human collaboration, clear communication forms the foundation of successful human-AI partnerships.
Robust Feedback Mechanisms
Agentic AI should provide meaningful feedback about its operations and make it easy for users to provide input on its performance. This bidirectional exchange enables:
- Continuous learning and refinement of the agent's behavior
- Adaptation to individual user preferences
- Improved accuracy and usefulness over time
The most effective agents make feedback feel conversational rather than mechanical, encouraging users to shape the AI's behavior through natural interaction.
Thoughtful Error Handling
How an autonomous agent handles mistakes significantly impacts user trust and satisfaction. Effective error handling includes:
- Proactively identifying potential errors before they occur
- Clearly communicating when and why errors happen
- Providing straightforward paths for recovery or human intervention
- Learning from mistakes to prevent recurrence
The ability to gracefully manage errors and learn from them is often what separates exceptional Agentic AI experiences from frustrating ones.
Appropriate User Control
Users need intuitive mechanisms to guide and control autonomous agents, including:
- Setting goals and parameters for the AI to work within
- The ability to pause or stop actions in progress
- Options to override decisions when necessary
- Preferences that persist across sessions
The level of control should adapt to both user expertise and task criticality, offering more granular options for advanced users or high-stakes decisions.
Balanced Transparency
Effective Agentic AI provides appropriate visibility into its reasoning and decision-making processes without overwhelming users. This involves:
- Making the AI's "thinking" visible and understandable
- Explaining data sources and how they influence decisions
- Offering progressive disclosure—basic explanations for casual users, deeper insights for those who want them
Transparency builds trust by demystifying what might otherwise feel like a "black box" of AI decision-making.
Proactive Assistance
Perhaps the most distinctive aspect of Agentic AI is its ability to anticipate needs and take initiative, offering:
- Relevant suggestions based on user context
- Automation of routine tasks without explicit commands
- Timely information that helps users make better decisions
When implemented thoughtfully, this proactive assistance transforms the AI from a passive tool into a true collaborative partner.
Building User Confidence Through Transparency and Explainability
For users to embrace autonomous agents, they need to understand and trust how these systems operate. This requires both transparency (being open about how the system works) and explainability (providing clear reasons for specific decisions).
Several techniques can enhance these critical qualities:
- Feature visualization that shows what the AI is "seeing" or focusing on
- Attribution methods that identify influential factors in decisions
- Counterfactual explanations that illustrate "what if" scenarios
- Natural language explanations that translate complex reasoning into simple terms
From a UX perspective, this means designing interfaces that:
- Clearly indicate when users are interacting with AI versus human systems
- Make complex decisions accessible through visualizations or natural language
- Offer progressive disclosure—basic explanations by default with deeper insights available on demand
- Implement audit trails documenting the AI's actions and reasoning
The goal is to provide the right information at the right time, helping users understand the AI's behavior without drowning them in technical details.
Embracing Iteration and Continuous Testing
The dynamic, learning nature of Agentic AI makes traditional "design once, deploy forever" approaches inadequate. Instead, successful development requires:
Iterative Design Processes
- Starting with minimal viable agents and expanding capabilities based on user feedback
- Incorporating user input at every development stage
- Continuously refining the AI's behavior based on real-world interaction data
Comprehensive Testing Approaches
- A/B testing different AI behaviors with actual users
- Implementing feedback loops for ongoing improvement
- Monitoring key performance indicators related to user satisfaction and task completion
- Testing for edge cases, adversarial inputs, and ethical alignment
Cross-Functional Collaboration
- Breaking down silos between UX designers, AI engineers, and domain experts
- Ensuring technical capabilities align with user needs
- Creating shared understanding of both technical constraints and user expectations
This ongoing cycle of design, testing, and refinement ensures Agentic AI continuously evolves to better serve user needs.
Learning from Real-World Success Stories
Several existing applications offer valuable lessons for designing effective autonomous systems:
Autonomous Vehicles demonstrate the importance of clearly communicating intentions, providing reassurance during operation, and offering intuitive override controls for passengers.
Smart Assistants like Alexa and Google Assistant highlight the value of natural language processing, personalization based on user preferences, and proactive assistance.
Robotic Systems in industrial settings showcase the need for glanceable information, simplified task selection, and workflows that ensure safety in shared human-robot environments.
Healthcare AI emphasizes providing relevant insights to professionals, automating routine tasks to reduce cognitive load, and enhancing patient care through personalized recommendations.
Customer Service AI prioritizes personalized interactions, 24/7 availability, and the ability to handle both simple requests and complex problem-solving.
These successful implementations share several common elements:
- They prioritize transparency about capabilities and limitations
- They provide appropriate user control while maximizing the benefits of autonomy
- They establish clear expectations about what the AI can and cannot do
Shaping the Future of Human-Agent Interaction
Designing user experiences for Agentic AI represents a fundamental shift in how we think about human-computer interaction. The evolution from graphical user interfaces to autonomous agents requires UX professionals to:
- Move beyond traditional design patterns focused on direct manipulation
- Develop new frameworks for building trust in autonomous systems
- Create interaction models that balance AI initiative with user control
- Embrace continuous refinement as both technology and user expectations evolve
The future of UX in this space will likely explore more natural interaction modalities (voice, gesture, mixed reality), increasingly sophisticated personalization, and thoughtful approaches to ethical considerations around AI autonomy.
For UX professionals and AI developers alike, this new frontier offers the opportunity to fundamentally reimagine the relationship between humans and technology—moving from tools we use to partners we collaborate with. By focusing on deep user understanding, transparent design, and iterative improvement, we can create autonomous AI experiences that genuinely enhance human capability rather than simply automating tasks.
The journey has just begun, and how we design these experiences today will shape our relationship with intelligent technology for decades to come.

Get Reliable Survey Results Fast: AI-Powered Question Simplification
At Optimal, we believe in the transformative potential of AI to accelerate your workflow and time to insights. Our goal is simple: keep humans at the heart of every insight while using AI as a powerful partner to amplify your expertise.
By automating repetitive tasks, providing suggestions for your studies, and streamlining workflows, AI frees you up to focus on what matters most—delivering impact, making strategic decisions, and building products people love.
That’s why we’re excited to announce our latest AI feature: AI-Powered Question Simplification.
Simplify and Refine Your Questions Instantly
Ambiguous or overly complex wording can confuse respondents, making it harder to get reliable, accurate insights. Plus, refining survey and question language is manual and can be a time-consuming process with little guidance. To solve this, we built an AI-powered tool to help study creators craft questions that resonate with participants and speed up the process of designing studies.
Our new AI-powered feature helps with:
- Instant Suggestions: Simplify complex question wording and improve clarity to make your questions easier to understand.
- Seamless Editing: Accept, reject, or regenerate suggestions with just a click, giving you complete control.
- Better Insights: By refining your questions, you’ll gather more accurate responses, leading to higher-quality data that drives better decisions.
Apply AI-Powered Question Simplification to any of your survey questions or to screening questions, and pre- and post-study questions in prototype tests, surveys, card sorts, tree tests, and first-click tests.
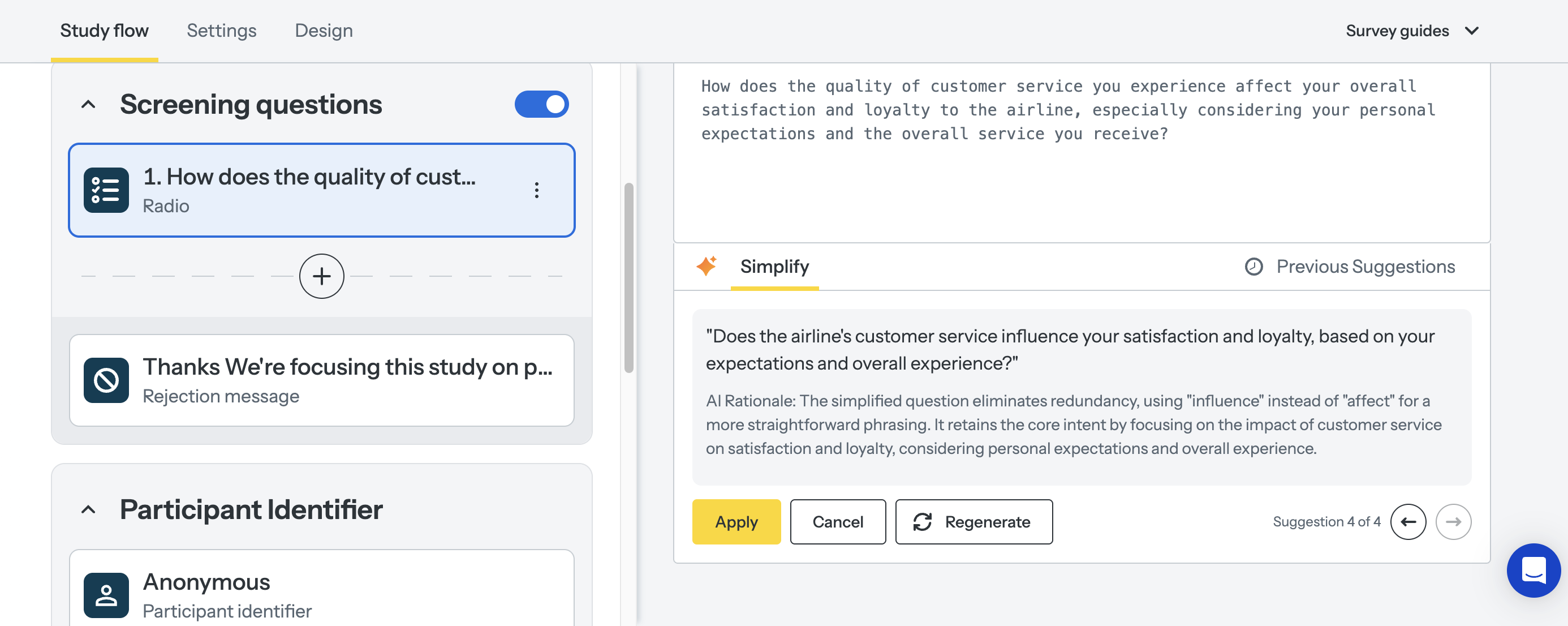
AI: Your Research Partner, Not a Replacement
AI is at the forefront of our innovation at Optimal this year, and we’re building AI into Optimal with clear principles in mind:
- AI does the tedious work: It takes on repetitive, mundane tasks, freeing you to focus on insights and strategy.
- AI assists, not dictates: You can adapt, change, or ignore AI suggestions entirely.
- AI is a choice: We recognize that Optimal users have diverse needs and risk appetites. You remain in control of how, when, and if you use AI.
A Growing Suite of AI-Powered Tools
The introduction of Question Simplification is just one example of how we’re leveraging AI to make research more efficient and effective for people who do research.
In 2024, we launched our AI Insights within our Qualitative Insights tool, summarizing key takeaways from interviews and transcripts. Now, we’re diving even deeper, exploring more ways to use AI to make research more efficient and effective.
Ready to Get Started?
Keep an eye out for more updates throughout 2025 as we continue to expand our platform with AI-powered features that help you uncover insights with speed, clarity, and more confidence.
Want to see how AI can speed up your workflow?
Apply AI-Powered Question Simplification today or check out AI Insights to experience it for yourself!

From Projects to Products: A Growing Career Trend
Introduction
The skills market has a familiar whiff to it. A decade ago, digital execs scratched their heads as great swathes of the delivery workforce decided to retrain as User Experience experts. Project Managers and Business Analysts decided to muscle-in on the creative process that designers insisted was their purview alone. Win for systemised thinking. Loss for magic dust and mystery.
With UX, research and design roles being the first to hit the cutting room floor over the past 24 months, a lot of the responsibility to solve for those missing competencies in the product delivery cycle now resides with the T-shaped Product Managers, because their career origin story tends to embrace a broader foundation across delivery and design disciplines. And so, as UX course providers jostle for position in a distracted market, senior professionals are repackaging themselves as Product Managers.
Another Talent Migration? We’ve Seen This Before.
The skills market has a familiar whiff to it. A decade ago, Project Managers (PMs) and Business Analysts (BAs) pivoted into UX roles in their droves, chasing the north star of digital transformation and user-centric design. Now? The same opportunities to pivot are emerging again—this time into Product Management.
And if history is anything to go by, we already know how this plays out.
Between 2015 and 2019, UX job postings skyrocketed by 320%, fueled by digital-first strategies and a newfound corporate obsession with usability. PMs and BAs, sensing the shift, leaned into their adjacent skills—stakeholder management, process mapping, and research—and suddenly, UX wasn’t just for designers anymore. It was a business function.
Fast-forward to 2025, and Product Management is in the same phase of maturation and despite some Covid-led contraction, bouncing back to 5.1% growth. The role has evolved from feature shipping to strategic value creation while traditional project management roles are trending towards full-stack product managers who handle multiple aspects of product development with fractional PMs for part-time or project-based roles.
Why Is This Happening? The Data Tells the Story.
📈 Job postings for product management roles grew by 41% between 2020 and 2025, compared to a 23% decline in traditional project management roles during the same period (Indeed Labor Market Analytics).
📉 The demand for product managers has been growing, with roles increasing by 32% yearly in general terms, as mentioned in some reports.
💰 Salary Shenanigans: Product Managers generally earn higher salaries than Business Analysts. In the U.S., PMs earn about 45% more than BAs on average ($124,000 vs. $85,400). In Australia, PMs earn about 4% to 30% more than BAs ($130,000 vs. $105,000 to $125,000) wave.
Three Structural Forces Driving the Shift
- Agile and Product-Led Growth Have Blurred the Lines
Project success is no longer measured in timelines and budgets—it’s about customer lifetime value (CLTV) and feature adoption rates. For instance, 86% of teams have adopted the Agile approach, and 63% of IT teams are also using Agile methodologies forcing PMs to move beyond execution into continuous iteration and outcome-based thinking.
- Data Is the New Currency, and BAs Are Cashing In
89% of product decisions in 2025 rely on analytics (Gartner, 2024). That’s prime territory for BAs, whose SQL skills, A/B testing expertise, and KPI alignment instincts make them critical players in data-driven product strategy.
- Role Consolidation Is Inevitable
The post-pandemic belt-tightening has left one role doing the job of three. Today’s product managers don’t just prioritise backlogs - they manage stakeholders, interpret data, and (sometimes poorly) sketch out UX wireframes. Product manager job descriptions now list "requirements gathering" and "stakeholder management"—once core PM/BA responsibilities.
How This Mirrors the UX Migration of 2019
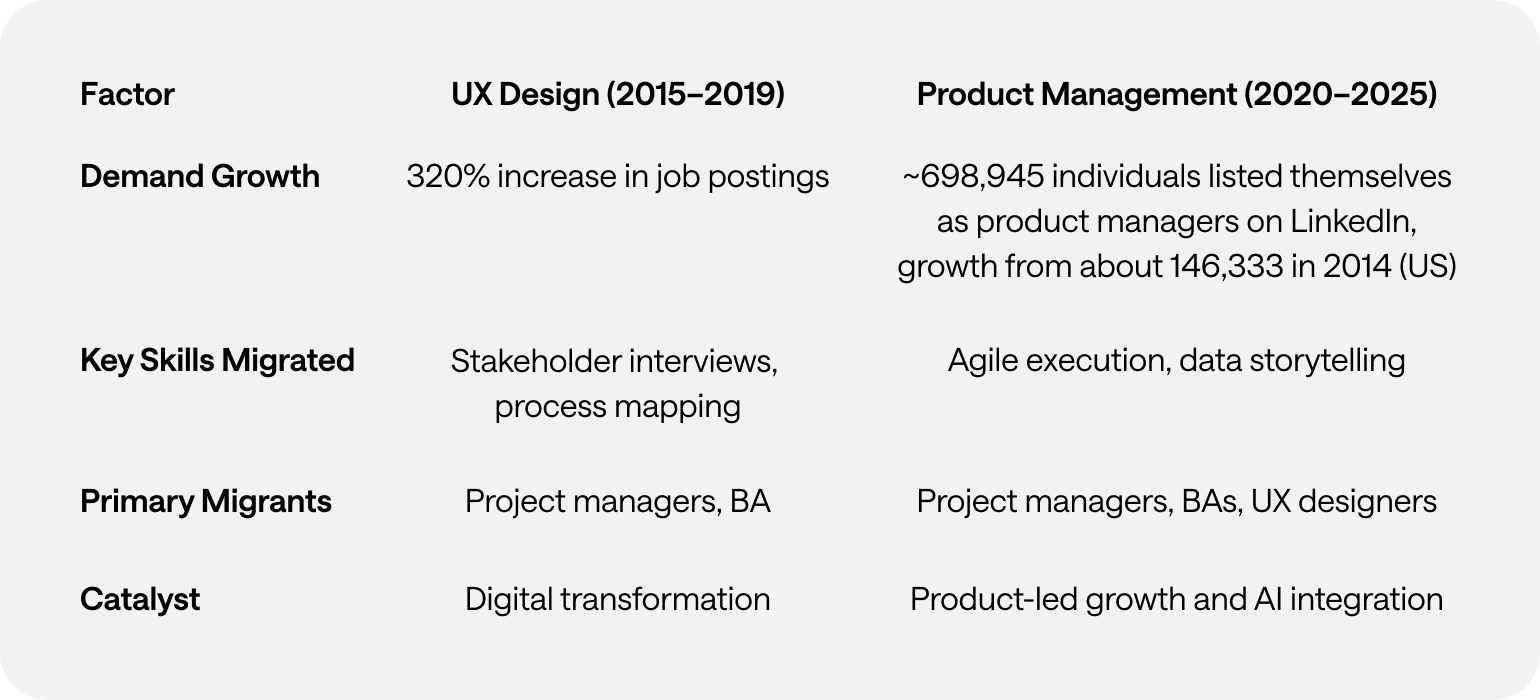
Same pattern. Different discipline.
The Challenges of Becoming a Product Manager (and Why Some Will Struggle)
👀 Outputs vs. Outcomes – PMs think in deliverables. Transitioning PMs struggle to adjust to measuring success through customer impact instead of project completion.
🛠️ Legacy Tech Debt – Outdated tech stacks can lead to decreased productivity, integration issues, and security concerns. This complexity can slow down operations and hinder the efficiency of teams, including product management.
😰 Imposter Syndrome is Real – New product managers feel unqualified, mirroring the self-doubt UX migrants felt in 2019. Because let’s be honest—jumping into product strategy is a different beast from managing deliverables.
What Comes Next? The Smartest Companies Are Already Preparing.
🏆 Structured Reskilling – Programs like Google’s "PM Launchpad" reduce time-to-proficiency for new PMs. Enterprises that invest in structured career shifts will win the talent war.
📊 Hybrid Role Recognition – Expect to see “Analytics-Driven PM” and “Technical Product Owner” job titles formalising this shift, much like “UX Strategist” emerged post-2019.
🚀 AI Will Accelerate the Next Migration – As AI automates routine PM/BA tasks, expect even more professionals to pivot into strategic product roles. The difference? This time, the transition will be even faster.
Conclusion: The Cycle Continues
Tech talent moves in cycles. Product Management is simply the next career gold rush for systems thinkers with a skill for structure, process, and problem-solving. A structural response to the evolution of tech ecosystems.
Companies that recognise and support this transition will outpace those still clinging to rigid org charts. Because one thing is clear—the talent migration isn’t coming. It’s already here.
This article was researched with the help of Perplexity.ai
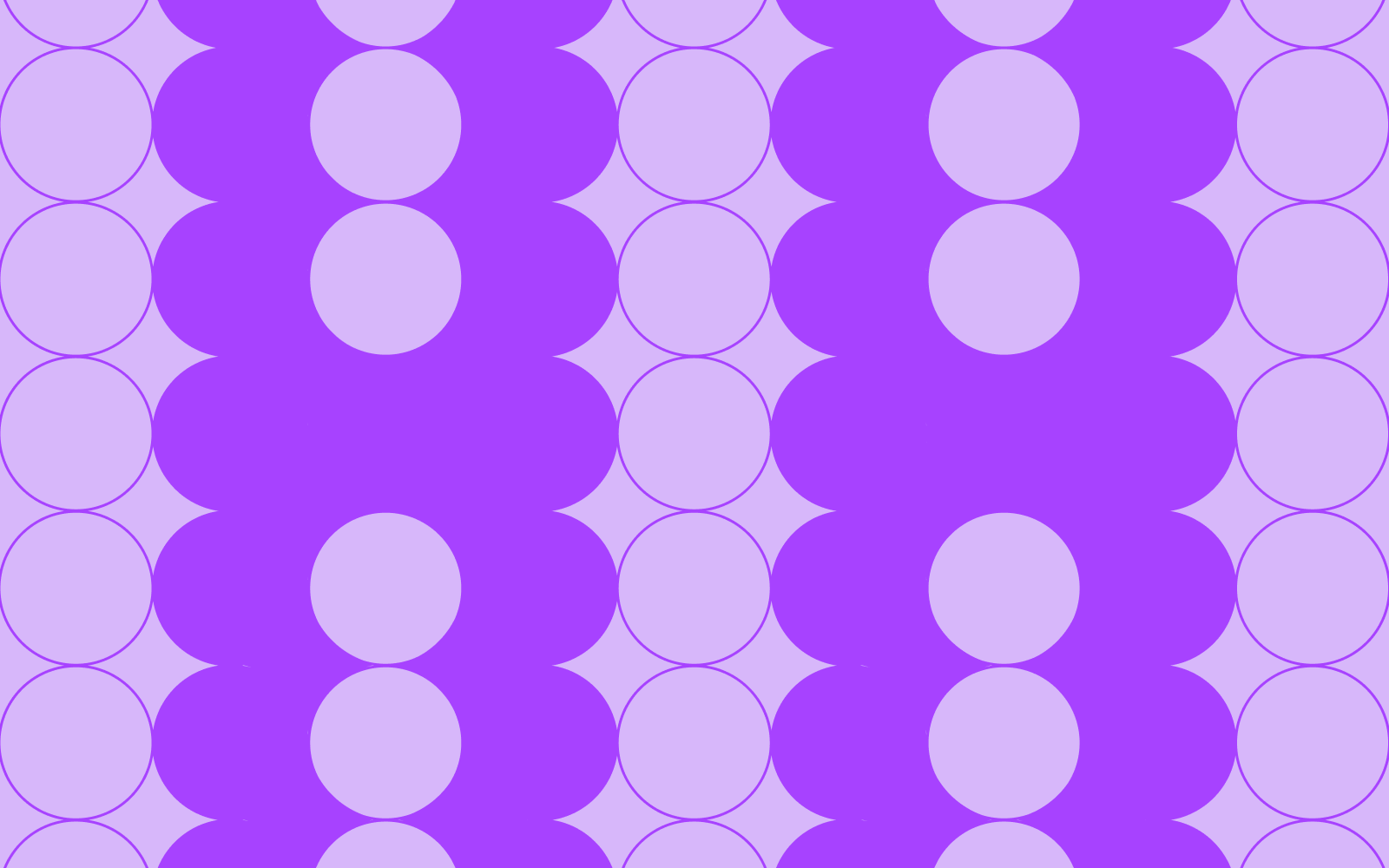
The Evolution of UX Research: Digital Twins and the Future of User Insight
Introduction
User Experience (UX) research has always been about people. How they think, how they behave, what they need, and—just as importantly—what they don’t yet realise they need. Traditional UX methodologies have long relied on direct human input: interviews, usability testing, surveys, and behavioral observation. The assumption was clear—if you want to understand people, you have to engage with real humans.
But in 2025, that assumption is being challenged.
The emergence of digital twins and synthetic users—AI-powered simulations of human behavior—is changing how researchers approach user insights. These technologies claim to solve persistent UX research problems: slow participant recruitment, small sample sizes, high costs, and research timelines that struggle to keep pace with product development. The promise is enticing: instantly accessible, infinitely scalable users who can test, interact, and generate feedback without the logistical headaches of working with real participants.
Yet, as with any new technology, there are trade-offs. While digital twins may unlock efficiencies, they also raise important questions: Can they truly replicate human complexity? Where do they fit within existing research practices? What risks do they introduce?
This article explores the evolving role of digital twins in UX research—where they excel, where they fall short, and what their rise means for the future of human-centered design.
The Traditional UX Research Model: Why Change?
For decades, UX research has been grounded in methodologies that involve direct human participation. The core methods—usability testing, user interviews, ethnographic research, and behavioral analytics—have been refined to account for the unpredictability of human nature.
This approach works well, but it has challenges:
- Participant recruitment is time-consuming. Finding the right users—especially niche audiences—can be a logistical hurdle, often requiring specialised panels, incentives, and scheduling gymnastics.
- Research is expensive. Incentives, moderation, analysis, and recruitment all add to the cost. A single usability study can run into tens of thousands of dollars.
- Small sample sizes create risk. Budget and timeline constraints often mean testing with small groups, leaving room for blind spots and bias.
- Long feedback loops slow decision-making. By the time research is completed, product teams may have already moved on, limiting its impact.
In short: traditional UX research provides depth and authenticity, but it’s not always fast or scalable.
Digital twins and synthetic users aim to change that.
What Are Digital Twins and Synthetic Users?
While the terms digital twins and synthetic users are sometimes used interchangeably, they are distinct concepts.
Digital Twins: Simulating Real-World Behavior
A digital twin is a data-driven virtual representation of a real-world entity. Originally developed for industrial applications, digital twins replicate machines, environments, and human behavior in a digital space. They can be updated in real time using live data, allowing organisations to analyse scenarios, predict outcomes, and optimise performance.
In UX research, human digital twins attempt to replicate real users' behavioral patterns, decision-making processes, and interactions. They draw on existing datasets to mirror real-world users dynamically, adapting based on real-time inputs.
Synthetic Users: AI-Generated Research Participants
While a digital twin is a mirror of a real entity, a synthetic user is a fabricated research participant—a simulation that mimics human decision-making, behaviors, and responses. These AI-generated personas can be used in research scenarios to interact with products, answer questions, and simulate user journeys.
Unlike traditional user personas (which are static profiles based on aggregated research), synthetic users are interactive and capable of generating dynamic feedback. They aren’t modeled after a specific real-world person, but rather a combination of user behaviors drawn from large datasets.
Think of it this way:
- A digital twin is a highly detailed, data-driven clone of a specific person, customer segment, or process.
- A synthetic user is a fictional but realistic simulation of a potential user, generated based on behavioral patterns and demographic characteristics.
Both approaches are still evolving, but their potential applications in UX research are already taking shape.
Where Digital Twins and Synthetic Users Fit into UX Research
The appeal of AI-generated users is undeniable. They can:
- Scale instantly – Test designs with thousands of simulated users, rather than just a handful of real participants.
- Eliminate recruitment bottlenecks – No need to chase down participants or schedule interviews.
- Reduce costs – No incentives, no travel, no last-minute no-shows.
- Enable rapid iteration – Get user insights in real time and adjust designs on the fly.
- Generate insights on sensitive topics – Synthetic users can explore scenarios that real participants might find too personal or intrusive.
These capabilities make digital twins particularly useful for:
- Early-stage concept validation – Rapidly test ideas before committing to development.
- Edge case identification – Run simulations to explore rare but critical user scenarios.
- Pre-testing before live usability sessions – Identify glaring issues before investing in human research.
However, digital twins and synthetic users are not a replacement for human research. Their effectiveness is limited in areas where emotional, cultural, and contextual factors play a major role.
The Risks and Limitations of AI-Driven UX Research
For all their promise, digital twins and synthetic users introduce new challenges.
- They lack genuine emotional responses.
AI can analyse sentiment, but it doesn’t feel frustration, delight, or confusion the way a human does. UX is often about unexpected moments—the frustrations, workarounds, and “aha” realisations that define real-world use.
- Bias is a real problem.
AI models are trained on existing datasets, meaning they inherit and amplify biases in those datasets. If synthetic users are based on an incomplete or non-diverse dataset, the research insights they generate will be skewed.
- They struggle with novelty.
Humans are unpredictable. They find unexpected uses for products, misunderstand instructions, and behave irrationally. AI models, no matter how advanced, can only predict behavior based on past patterns—not the unexpected ways real users might engage with a product.
- They require careful validation.
How do we know that insights from digital twins align with real-world user behavior? Without rigorous validation against human data, there’s a risk of over-reliance on synthetic feedback that doesn’t reflect reality.
A Hybrid Future: AI + Human UX Research
Rather than viewing digital twins as a replacement for human research, the best UX teams will integrate them as a complementary tool.
Where AI Can Lead:
- Large-scale pattern identification
- Early-stage usability evaluations
- Speeding up research cycles
- Automating repetitive testing
Where Humans Remain Essential:
- Understanding emotion, frustration, and delight
- Detecting unexpected behaviors
- Validating insights with real-world context
- Ethical considerations and cultural nuance
The future of UX research is not about choosing between AI and human research—it’s about blending the strengths of both.
Final Thoughts: Proceeding With Caution and Curiosity
Digital twins and synthetic users are exciting, but they are not a magic bullet. They cannot fully replace human users, and relying on them exclusively could lead to false confidence in flawed insights.
Instead, UX researchers should view these technologies as powerful, but imperfect tools—best used in combination with traditional research methods.
As with any new technology, thoughtful implementation is key. The real opportunity lies in designing research methodologies that harness the speed and scale of AI without losing the depth, nuance, and humanity that make UX research truly valuable.
The challenge ahead isn’t about choosing between human or synthetic research. It’s about finding the right balance—one that keeps user experience truly human-centered, even in an AI-driven world.
This article was researched with the help of Perplexity.ai.
.webp)
Product Update - March 2025
2025 is already proving to be an exciting year for UX research, and we’re just getting started! With a range of new features and updates that empower teams to move from design to decisions faster, Optimal is bringing the best tools to the table for everyone and making research accessible across all teams. Let’s dive into what’s already here and what's coming next!
Video Recording for Prototype Testing
We’re excited to announce that the closed beta for video recording for prototype testing has launched. By capturing screen, audio, and/or video, this feature takes research beyond metrics to give you a deeper understanding of user intent and pain points. Our Optimal Recruitment service also ensures you connect with the right participants for video recording, driving meaningful insights from the start.
New Study Flow for Faster Study Creation
Say hello to the new Study Flow, an intuitive tab that helps to accelerate study creation, combining the Messages & instructions and Questionnaire tabs into one. Visualize every step of the participant’s journey, from the welcome screen to the final thank-you message with the Study Flow panel. Save time by quickly duplicating questions across Surveys and other study types. Navigate through studies with ease by collapsing and expanding sections as needed.
Dashboard & Templates Library
We’re working to make research more accessible for everyone with the release of 15+ new templates designed to help Optimal users get started quickly. No matter which team you’re on, these use-case focused, ready-to-use templates will set you on the fast track. Use them to preview the participant experience or clone the recommended study and tailor for your own use.
What We’re Working On Next
Here’s a sneak peek at some exciting features in the works:
AI-Powered Survey Question Simplification
We’re unleashing an AI-powered feature to help users simplify complex question wording and improve clarity. Users can quickly accept, reject, or regenerate more suggestions with a click. By improving clarity and simplifying your questions, you’ll gather more accurate, higher-quality insights that drive better results.
This AI feature is available for surveys questions as well as screening, pre and post study questions for surveys, prototype tests, card sorts, tree tests and first-click tests. This is just the beginning of even more AI-driven improvements to come, all aimed at helping to accelerate your time to insights.
Advanced Logic Capabilities
We’re working on bringing more advanced logic capabilities to Optimal - one of the most highly requested features for surveys. With display logic, the study changes dynamically - showing or hiding answer options or subsequence questions based on a participant’s previous responses. Apply display logic to Surveys, screening questions, and pre- and post-study questions. This is just the beginning. We’ll be exploring additional advanced logic capabilities in 2025.
Study Creation Enhancements
Optimal will continue to improve on the new Study Flow to make study creation faster and intuitive. You’ll also gain greater flexibility with customizable sections. These upcoming updates are designed to empower you to create dynamic, tailored study experiences for different audiences, helping you unlock more valuable insights with ease.
With custom sections, you’ll be able to organize surveys and questions into distinct sections, such as demographics or product usage, to create a more structured and engaging study. These custom sections allow you to easily add, rename, reorder, duplicate, and move questions between sections.
Join Us on the Journey
Stay tuned for regular updates, and let us know how we can make your research experience even better. Have feedback or feature requests? We’d love to hear from you so we can continue to shape the future of Optimal.

Accelerate Study Creation with the New Study Flow
Inspired by insights from Optimal users, we’ve reimagined study creation to bring you a beautifully streamlined experience with the new Study Flow tab.
With the new Study Flow, you’ll:
⚡ Enjoy faster study set up: Messages & instructions and Questionnaire tabs are combined in a single tab - called Study Flow - for faster editing and settings customization.
✨ See it all at a glance: Easily visualize and understand the participant experience—from the welcome message to the final thank-you screen—every step of the way.
🎯 Duplicate questions: Save time and quickly replicate questions for surveys, screening questions, and pre- and post-study questions.
⭐ Experience enhanced UI: Enjoy a modern, clean design with intuitive updates that minimize scrolling and reduce mental load.
🗂️ Collapse and expand sections: Easily navigate studies by collapsing and expanding sections, making it easier to build out specific parts of your study.
This Study Flow tab is available across all Optimal tools, except for Qualitative Insights.
What’s next?
We’re not stopping there. We have some significant improvements on the horizon designed to give you even greater flexibility and control.
Advanced logic: Enhanced logic capabilities is one of our most highly requested features, and we’re thrilled to introduce new capabilities to help you build your ideal study experience – available for surveys and other tools. We will first introduce “display logic”, allowing for:
- If answer is X for Question Y, then hide/show Question Z.
- If answer is X for Question Y, then hide/show specific answer options.
Customizable sections: Organize your questions into different sections to build a better study experience for your participants. For example, segment your questions into relevant groupings, such as demographics or product usage. With custom sections, you can add new sections, rename, reorder, duplicate, and move questions between different sections.*
*Note: Questions cannot be moved to/from the screening questions section.
These upcoming features will empower you to create dynamic, tailored study experiences for different audiences with ease for more valuable insights.
Start exploring the new Study Flow now.