Cards have been created, sorted and sorted again. The participants are all finished and you’re left with a big pile of awesome data that will help you improve the user experience of your information architecture. Now what?Whether you’ve run an open, hybrid or closed card sort online using an information architecture tool or you’ve run an in person (moderated) card sort, it can be a bit daunting trying to figure out where to start the card sort analysis process.
About this guide
This two-part guide will help you on your way! For Part 1, we’re going to look at how to interpret and analyze the results from open and hybrid card sorts.
- In open card sorts, participants sort cards into categories that make sense to them and they give each category a name of their own making.
- In hybrid card sorts, some of the categories have already been defined for participants to sort the cards into but they also have the ability to create their own.
Open and hybrid card sorts are great for generating ideas for category names and labels and understanding not only how your users expect your content to be grouped but also what they expect those groups to be called.In both parts of this series, I’m going to be talking a lot about interpreting your results using Optimal Workshop’s online card sorting tool, OptimalSort, but most of what I’m going to share is also applicable if you’re analyzing your data using a spreadsheet or using another tool.
Understanding the two types of analysis: exploratory and statistical
Similar to qualitative and quantitative methods, exploratory and statistical analysis in card sorting are two complementary approaches that work together to provide a detailed picture of your results.
- Exploratory analysis is intuitive and creative. It’s all about going through the data and shaking it to see what ideas, patterns and insights fall out. This approach works best when you don’t have the numbers (smaller sample sizes) and when you need to dig into the details and understand the ‘why’ behind the statistics.
- Statistical analysis is all about the numbers. Hard data that tells you exactly how many people expected X to be grouped with Y and more and is very useful when you’re dealing with large sample sizes and when identifying similarities and differences across different groups of people.
Depending on your objectives - whether you are starting from scratch or redesigning an existing IA - you’ll generally need to use some combination of both of these approaches when analyzing card sort results. Learn more about exploratory and statistical analysis in Donna Spencer’s book.
Start with the big picture
When analyzing card sort results, start by taking an overall look at the results as a whole. Quickly cast your eye over each individual card sort and just take it all in. Look for common patterns in how the cards have been sorted and the category names given by participants. Does anything jump out as surprising? Are there similarities or differences between participant sorts? If you’re redesigning an existing IA, how do your results compare to the current state?If you ran your card sort using OptimalSort, your first port of call will be the Overview and Participants Table presented in the results section of the tool.If you ran a moderated card sort using OptimalSort’s printed cards, now is a good time to double check you got them all. And if you didn’t know about this handy feature of OptimalSort, it’s something to keep in mind for next time!The Participants Table shows a breakdown of your card sorting data by individual participant. Start by reviewing each individual card sort one by one by clicking on the arrow in the far left column next to the Participants numbers.
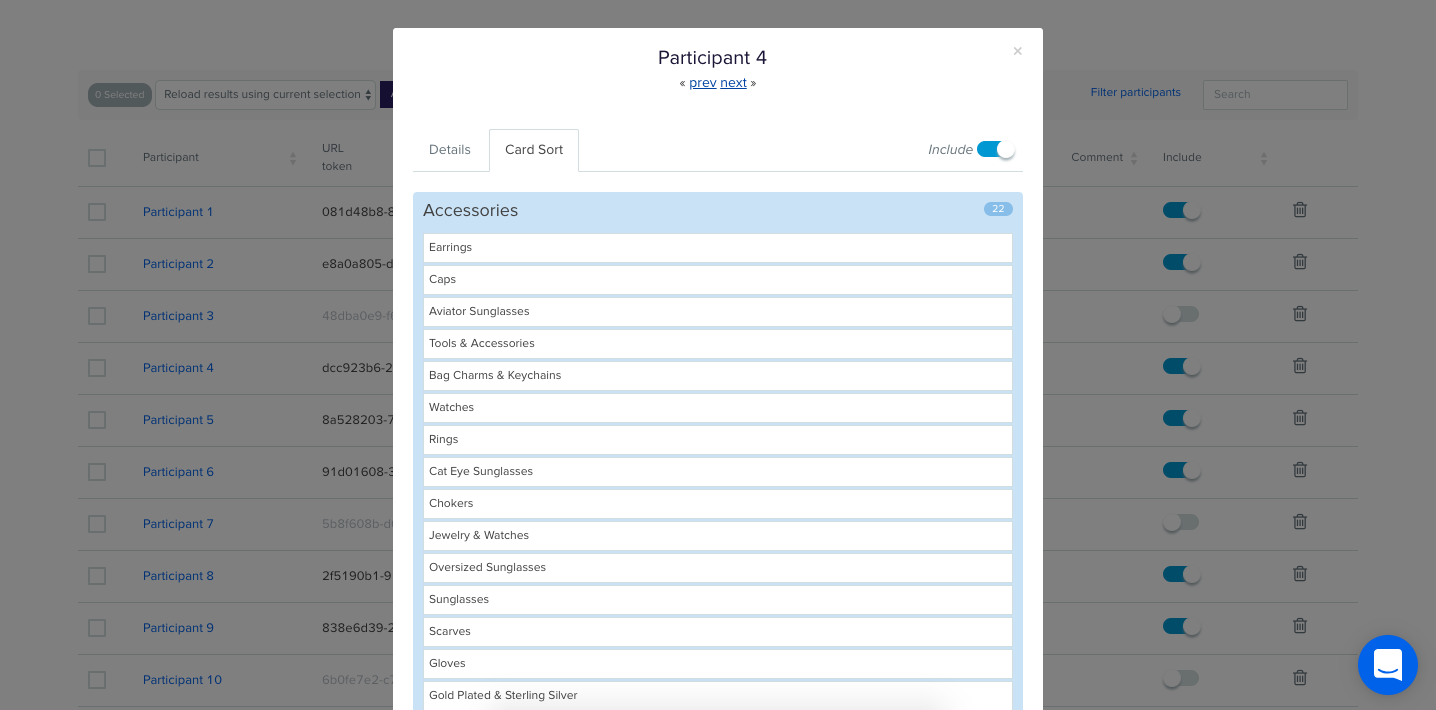
From here you can easily flick back and forth between participants without needing to close that modal window. Don’t spend too much time on this — you’re just trying to get a general impression of what happened.Keep an eye out for any card sorts that you might like to exclude from the results. For example participants who have lumped everything into one group and haven’t actually sorted the cards. Don’t worry - excluding or including participants isn’t permanent and can be toggled on or off at anytime.If you have a good number of responses, then the Participant Centric Analysis (PCA) tab (below) can be a good place to head next. It’s great for doing a quick comparison of the different high-level approaches participants took when grouping the cards.The PCA tab provides the most insight when you have lots of results data (30+ completed card sorts) and at least one of the suggested IAs has a high level of agreement among your participants (50% or more agree with at least one IA).
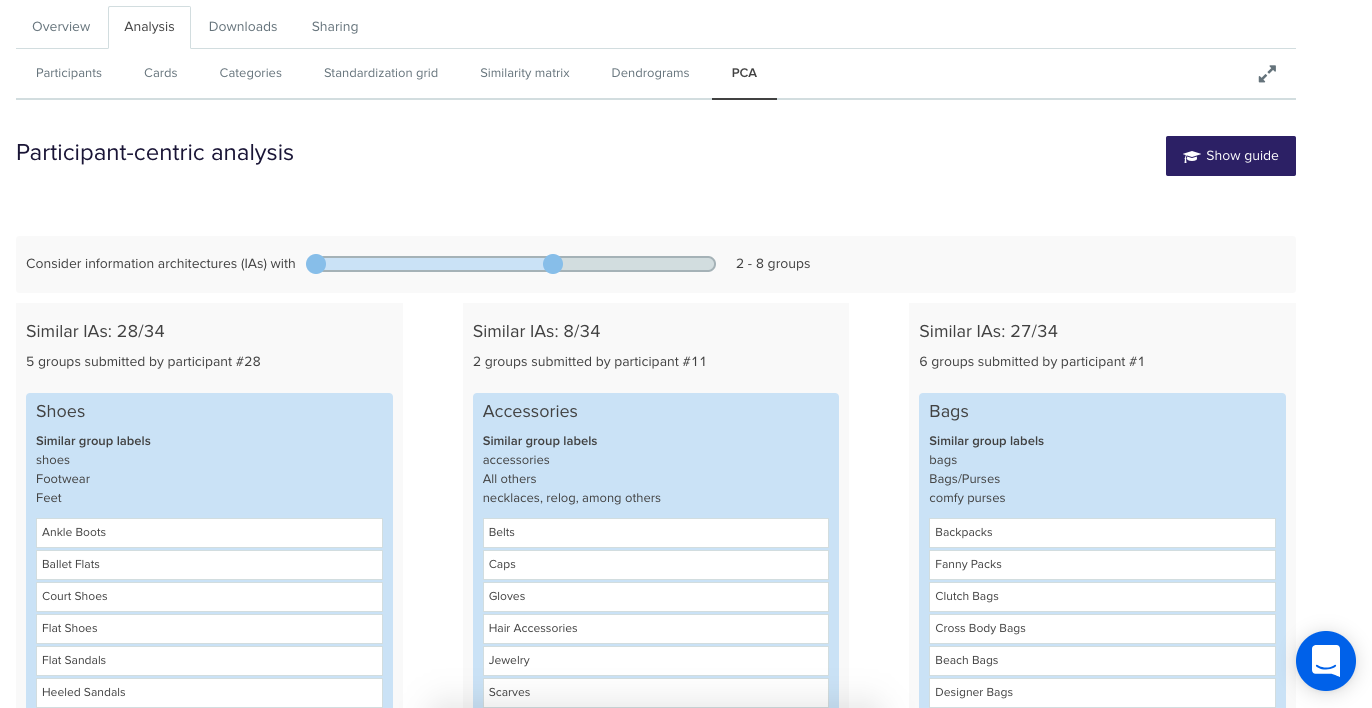
The PCA tab compares data from individual participants and surfaces the top three ways the cards were sorted. It also gives you some suggestions based on participant responses around what these categories could be called but try not to get too bogged down in those - you’re still just trying to gain an overall feel for the results at this stage.Now is also a good time to take a super quick peek at the Categories tab as it will also help you spot patterns and identify data that you’d like to dive deeper into a bit later on!Another really useful visualization tool offered by OptimalSort that will help you build that early, high-level picture of your results is the Similarity Matrix. This diagram helps you spot data clusters, or groups of cards that have been more frequently paired together by your participants, by surfacing them along the edge and shading them in dark blue. It also shows the proportion of times specific card pairings occurred during your study and displays the exact number on hover (below).
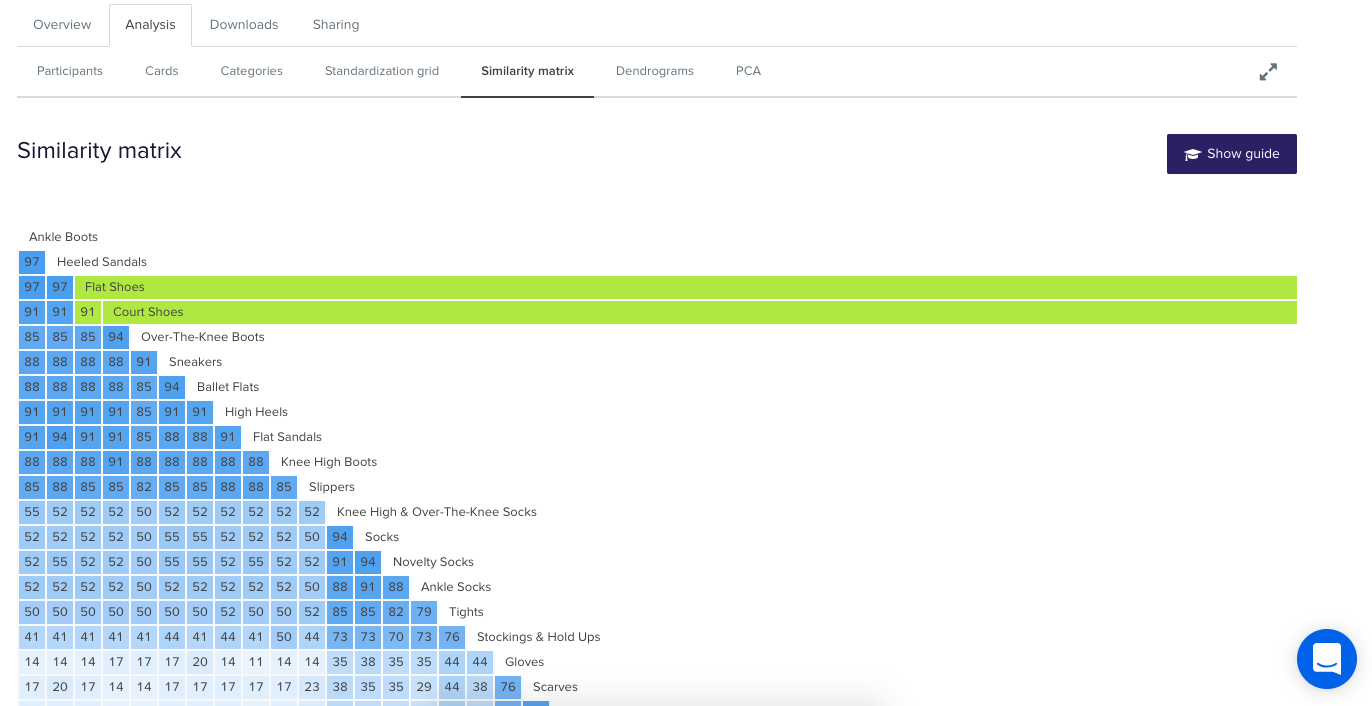
In the above screenshot example we can see three very clear clusters along the edge: ‘Ankle Boots’ to ‘Slippers’ is one cluster, ‘Socks’ to ‘Stockings & Hold Ups’ is the next and then we have ‘Scarves’ to ‘Sunglasses’. These clusters make it easy to spot the that cards that participants felt belonged together and also provides hard data around how many times that happened.Next up are the dendrograms. Dendrograms are also great for gaining an overall sense of how similar (or different) your participants’ card sorts were to each other. Found under the Dendrogram tab in the results section of the tool, the two dendrograms are generated by different algorithms and which one you use depends largely on how many participants you have.
If your study resulted in 30 or more completed card sorts, use the Actual Agreement Method (AAM) dendrogram and if your study had fewer than 30 completed card sorts, use the Best Merge Method (BMM) dendrogram.The AAM dendrogram (see below) shows only factual relationships between the cards and displays scores that precisely tell you that ‘X% of participants in this study agree with this exact grouping’.In the below example, the study shown had 34 completed card sorts and the AAM dendrogram shows that 77% of participants agreed that the cards highlighted in green belong together and a suggested name for that group is ‘Bling’. The tooltip surfaces one of the possible category names for this group and as demonstrated here it isn’t always the best or ‘recommended’ one. Take it with a grain of salt and be sure to thoroughly check the rest of your results before committing!

The BMM dendrogram (see below) is different to the AAM because it shows the percentage of participants that agree with parts of the grouping - it squeezes the data from smaller sample sizes and makes assumptions about larger clusters based on patterns in relationships between individual pairs.The AAM works best with larger sample sizes because it has more data to work with and doesn’t make assumptions while the BMM is more forgiving and seeks to fill in the gaps.The below screenshot was taken from an example study that had 7 completed card sorts and its BMM dendrogram shows that 50% of participants agreed that the cards highlighted in green down the left hand side belong to ‘Accessories, Bottoms, Tops’.

Drill down and cross-reference
Once you’ve gained a high level impression of the results, it’s time to dig deeper and unearth some solid insights that you can share with your stakeholders and back up your design decisions.Explore your open and hybrid card sort data in more detail by taking a closer look at the Categories tab. Open up each category and cross-reference to see if people were thinking along the same lines.Multiple participants may have created the same category label, but what lies beneath could be a very different story. It’s important to be thorough here because the next step is to start standardizing or chunking individual participant categories together to help you make sense of your results.In open and hybrid sorts, participants will be able to label their categories themselves. This means that you may identify a few categories with very similar labels or perhaps spelling errors or different formats. You can standardize your categories by merging similar categories together to turn them into one.OptimalSort makes this really easy to do - you pretty much just tick the boxes alongside each category name and then hit the ‘Standardize’ button up the top (see below). Don’t worry if you make a mistake or want to include or exclude groupings; you can unstandardize any of your categories anytime.
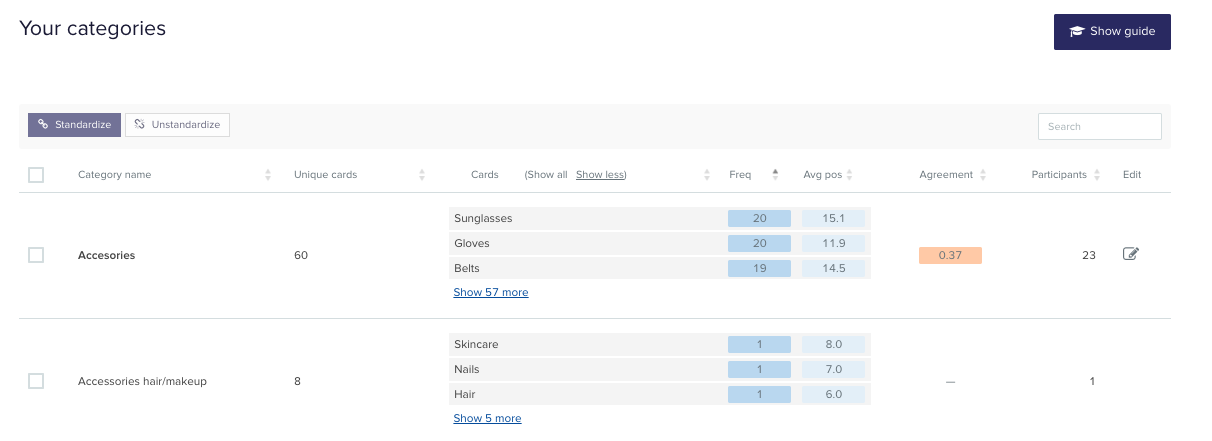
Once you’ve standardized a few categories, you’ll notice that the Agreement number may change. It tells you how many participants agreed with that grouping. An agreement number of 1.0 is equal to 100% meaning everyone agrees with everything in your newly standardized category while 0.6 means that 60% of your participants agree.Another number to watch for here is the number of participants who sorted a particular card into a category which will appear in the frequency column in dark blue in the right-hand column of the middle section of the below image.

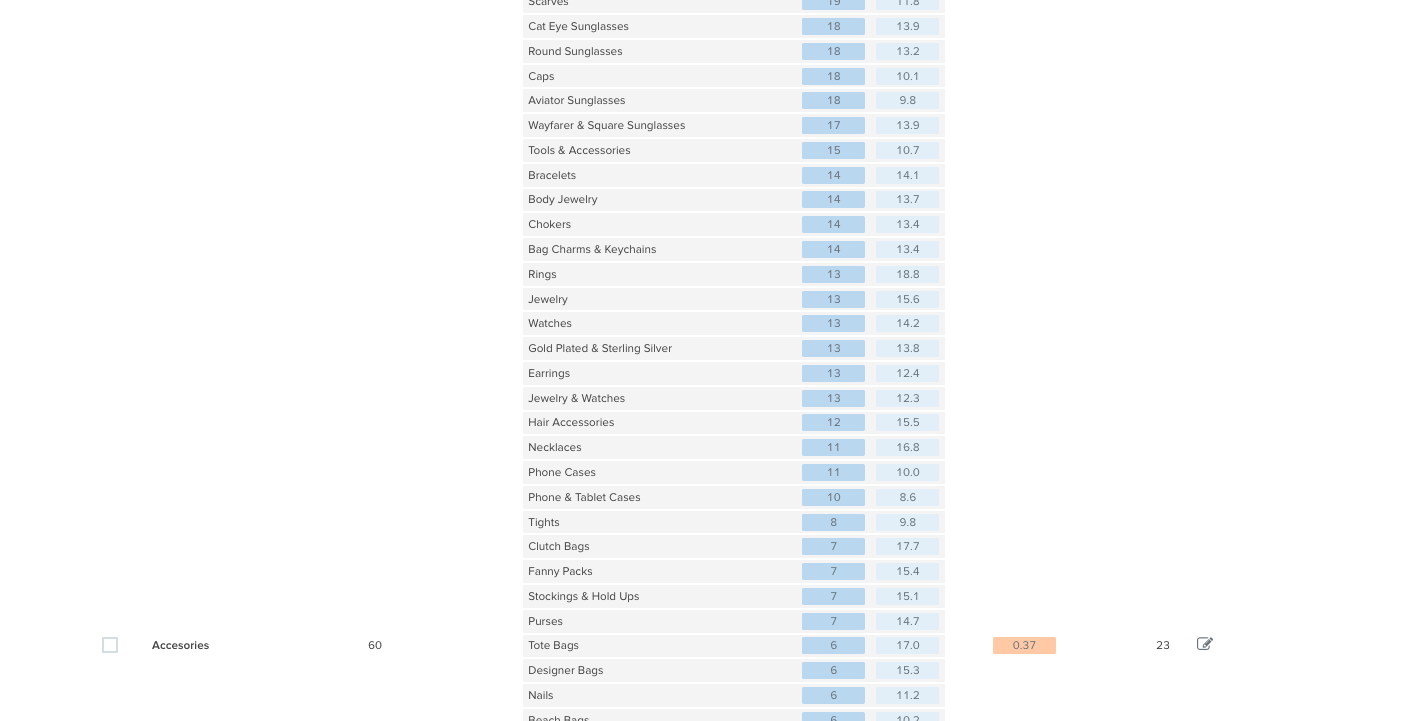
From the above screenshot we can see that in this study, 18 of the 26 participant categories selected agree that ‘Cat Eye Sunglasses’ belongs under ‘Accessories’.Once you’ve standardized a few more categories you can head over to the Standardization Grid tab to review your data in more detail. In the below image we can see that 18 participants in this study felt that ‘Backpacks’ belong in a category named ‘Bags’ while 5 grouped them under ‘Accessories’. Probably safe to say the backpacks should join the other bags in this case.
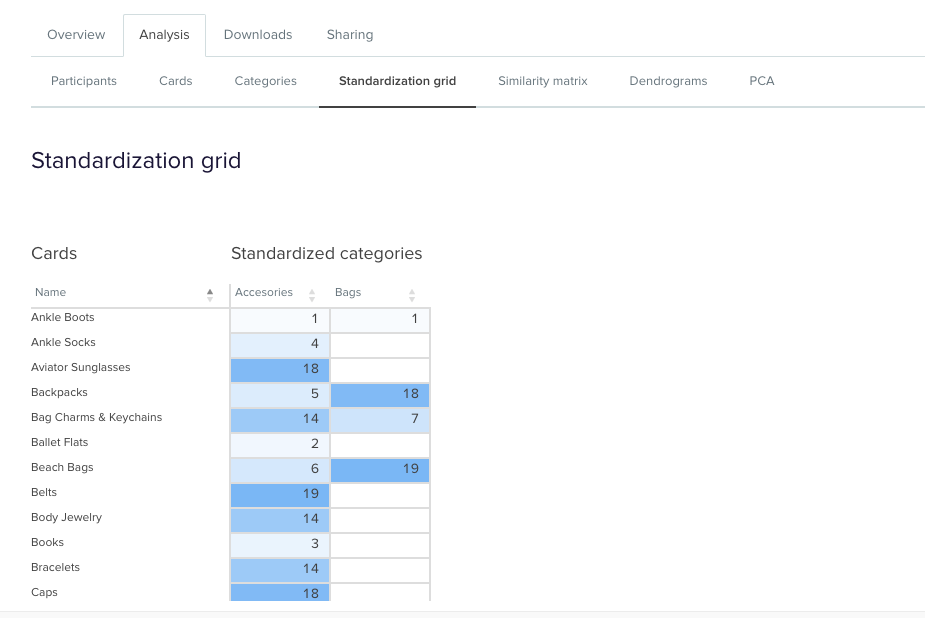
So that’s a quick overview of how to interpret the results from your open or hybrid card sorts.Here's a link to Part 2 of this series where we talk about interpreting results from closed card sorts as well as next steps for applying these juicy insights to your IA design process.
Further reading
- Card Sorting 101 – Learn about the differences between open, closed and hybrid card sorts, and how to run your own using OptimalSort.
- Which comes first: card sorting or tree testing? – Tree testing and card sorting can give you insight into the way your users interact with your site, but which comes first?
- How to pick cards for card sorting – What should you put on your cards to get the best results from a card sort? Here are some guidelines.